Last updated on January 17th, 2024 at 07:39 am
In the current business environment, artificial intelligence (AI), machine learning (ML), and deep learning (DL) are highly used technologies. Hence, the company uses these technologies to develop smart applications. Although these technologies are often used in business communication across the globe, many people face challenges in applying them. So, in this blog on AI vs ML Vs DL, we will discuss all essential points.
Here’s an example showing how machine learning, deep learning, and artificial intelligence are all significantly different.
Source: simplilearn.com
The concept of developing intelligent robots is known as “artificial intelligence.”
A concept called “machine learning” makes it easier for people to make apps that use artificial intelligence.
DL is a type of machine learning that uses advanced algorithms and multi-layer processing to pull high-level features out of data.
Let’s now look more closely at all of these technologies.
What is Artificial intelligence?
Artificial intelligence (AI) is illustrated as machine-based intelligence that simulates human behaviour or thinking. It can be trained to solve particular issues. AI is the combination of ML and DL techniques.
Types of artificial intelligence models are introduced using a more extensive data set. It has the competency to make intelligent decisions.
AI can also be illustrated as developing computer systems that are competent to perform the task. It needs human intelligence like object detection, decision-making, and solving challenging issues.
Data, information, and human cognition are transferred to computers via AI. The key aim of AI is to build automated systems with human-like thought processes.
These machines may work by learning and solving problems, mimicking human behaviour. Most artificial intelligence (AI) technologies mimic human intellect when handling complex issues.
Let’s explore the Amazon Echo as an example of an AI-powered device.
Alexa is an artificial intelligence (AI) virtual assistant that Amazon Echo, a smart speaker, utilizes. Amazon Alexa can talk to you, play music, set alarms, play podcasts, and give you accurate news, weather, sports, and traffic updates.
As seen in the graph below, someone is curious about the current temperature in Chicago. Firstly, the voice of the speaker is transformed into a machine-readable structure.
The Amazon Alexa system subsequently receives the structured data for analysis and manipulation. Lastly, Alexa uses the Amazon Echo to provide the necessary voice output.
After a brief overview of the fundamentals of artificial intelligence, let’s look at examples of each category.
Types of Artificial Intelligence
It can be classified into the following types based on the functionality of AI-based systems:
1. AI Reactive Machines
This kind of AI considers the present system and machines that operate simply on current data. Reactive AI systems can’t put together the data interactions needed to figure out what they might do in the future.
They are only capable of a limited set of predetermined movements. The IBM chess program that defeated the world champion is an example of reactive AI.
2. AI Limited Memory
By evaluating knowledge from the past that is stored in its memory, it can make more informed and better judgments. For instance, AI may be said to have a transient or short-lived memory that is used for storing prior events and, as a result, assessing possible deeds.
Autonomous vehicles are classified as AI with limited memory. It leverages the data that has recently been obtained to make quick judgments. To make smart driving decisions, self-driving cars utilize sensors to detect pedestrians crossing the road, traffic lights, and steep routes. It helps in avoiding potential blunders.
3. AI Theory of Mind
It is a very sophisticated kind of AI. It is thought that devices of this kind will significantly impact psychology. This kind of AI would strongly emphasize emotional intelligence; hence, human ideas and perspectives may be successfully understood.
Although it is not yet highly developed, serious research is being done in this field.
Self-Aware AI
Let’s strongly recommend against advancing AI to the point where computers become self-aware and have their own consciousness. Given the state of affairs right now, this type of AI is not entirely implausible.
But over time, reaching a level of super intelligence could be feasible. Elon Musk and Stephen Hawkings, two geniuses, have constantly warned us about the development of artificial intelligence.
Applications of Artificial Intelligence
Presently, AI is implemented in different manners. It is suitably significant in this advanced tech era because it can competently deal with complex challenges in various areas.
These areas are defence, robotics, transport, marketing, healthcare marketing, business, banking, gaming, and chatbots. Our daily lives are becoming highly efficient and convenient as a result of different applications of artificial intelligence.
Before going further, let us focus on the applications of AI in this blog:
Source: Simplilearn
Let us now look at AI applications in robotics.
1. AI Applications in Robotics
Robots that can do tasks on their own are the focus of the robotics industry. Nowadays, robots are highly efficient and advanced in meeting functions without human intervention. because AI techniques and tools are primarily aimed at the robotics industry.
Advanced robots contain voice recognition systems, high-definition cameras, and sensors.
These robots are competent at learning from previous errors and also experiencing and adjusting to the algorithms as per the environment. It is a highly efficient technique for robotic applications. While it is integrated with advanced devices, it can support optimizations.
These are supportive of improving the challenging manufacturing procedures in aerospace-related sectors. The packaging procedure in industries also uses artificial intelligence to improve productivity and lower the overall cost.
2. AI Applications in Healthcare
Nowadays, most healthcare organizations use AI-based technologies for their routine operations. These duties range from managing hospital data to patient diagnostics.
The amount of data generated by the healthcare industry daily is 44 trillion gigabytes. Therefore, there is a need for powerful processors powered by AI that can manipulate, extract, develop, and analyse the thorough insights from this data.
ML and AI technologies are performing better in the healthcare sector. The AI-based algorithms created for the systems are capable of detecting patterns much more accurately than humans.
These algorithms also support the assessment of patient data. Thus, it is supported by the diagnosis. AI-based systems aid in determining real-time data like body temperature, blood pressure, heartbeat, and much more.
They also send the gathered information to doctors to assess patients’ health conditions from remote locations. It can save time for both patients and doctors.
By streamlining the procedure, AI-based assistants and bots make doctors competent at keeping and managing patients’ time. Moreover, the time and locations of AI in healthcare support pathologists in the assessment of tissues and genes. It makes the diagnosis of certain diseases more feasible.
3. AI Applications in Marketing
A success factor for each business is marketing. Good marketing strategies lead to producing higher profits. As per Forbes, the revenue created by the marketing sector in 2019 was over US$300 million.
AI apps are currently transforming the marketing industry. Different sectors use AI to enhance profitability, like eCommerce, eLearning, advertising, e-wallet, media, and entertainment.
For example, when you search for a product on Amazon, similar products, best sellers, and recommended product lists will appear to you.
You may have noticed that when you search for a product, you get an ad for similar products. For instance, when you search for a particular Netflix series, you will get ads and recommendations for other shows. It is attained when AI-based ad algorithms try to satisfy the customer’s interests.
Furthermore, they improve and learn their performance over time. This automated marketing supports providing a customized experience to customers, targeting advertising, and improving the business’s revenue.
4. AI Applications in Gaming
AI has been a significant element of the gaming sector in recent years.
The gaming sector is the best example of intelligent AI because many changes are required to reach the end goal of this platform. AI is considered in different ways, such as in character development and game creation. Under gaming, AI focuses on adaptable and interactive video game experiences.
Non-player characters (NPCs) are used to create this type of AI-powered unique entertainment. It is also performed artistically and intellectually because the human player directs it. The AI engine helps assess how an NPC acts in the game environment.
5. AI Applications in Chatbots
It understands natural language and talks back to people who use live chat services that businesses offer to help with customer service.ML makes intelligent AI chatbots that can be integrated into various apps and websites.
Despite collecting data through the current option of inclusive responses, AI chatbots can develop a database of reactions. These chatbots can effectively evaluate customer issues, respond to fundamental studies, improve customer services, and offer 24×7 support as AI advances.
Hence, AI chatbots support improving customer satisfaction.
Also Read : Why Internet of Things Needs Artificial Intelligence?
How Does Artificial Intelligence Work?
The automated shifting of appliances at home is a typical use of AI that we see nowadays.
The detectors in the room recognize your presence and switch on the lights whenever you enter a dark environment. It is an illustration of a machine without memory. One of the more sophisticated AI algorithms may even estimate your consumption patterns and activate appliances without your direct input.
Some artificial intelligence (AI) algorithms can recognize your speech and respond appropriately. The TV will turn on when you command it using its sound sensors.
You can perform this daily with a Google Home Mini and a Google dongle.
The application of AI in healthcare is covered in the last part of this course on artificial intelligence.
What is Machine Learning?
In the subfield of computer science called “machine learning,” analytics and computer algorithms are used to make prediction models that help solve business problems.
McKinsey & Co. says that the foundation of ML is algorithms that could pull information out of information without using rules-based coding.
So, you can see many ways to define machine learning. But how exactly does it operate?
Types of Machine Learning
ML algorithms are divided into three major categories:
1. Supervised Learning
Since supervised learning has already categorized the information, you are informed about the target variable. Using this learning method, systems may predict future outcomes using previous information. For the model to be developed, a minimum of one insight and one outcome variable should be used.
The example below is a good example of supervised learning at work. Data from identified cats and dogs is used to construct the algorithm. It aids in assessing whether this model predicts the new image of a dog or a cat.
Supervised learning is used in regression analysis, Naive Bayes, nonlinear regression, decision trees, and support vector machines, among other things.
2. Unsupervised Learning
It employs unlabeled data to find patterns among the information dynamically. From the provided raw data, the systems may extract hidden properties. If the data is better understood, patterns and linkages may be seen more clearly.
The unsupervised learning technique used to train a model with unlabeled data is shown here. The information, in this case, comprised several vehicles. The model’s goal is to identify various vehicle types.
Unsupervised learning is used in the detection system, clustering algorithms, and k-means clustering, among other things.
3. Reinforcement Learning
An agent may learn how to complete a job in the context of uncertainty using reinforcement learning. The agent provides entertainment to the neighbourhood while gaining knowledge and benefits. The reward assesses how effectively a behaviour aids in achieving the task’s goal.
Here is an illustration of how a computer is taught to recognize shapes.
Deep Q-learning and Q-learning are good reinforcement learning models, including neural networks.
Applications of Machine Learning
ML is a modern technological term that is rapidly growing each day. We use machine learning in our daily lives without even realizing it: Google Assistant, Google Maps, and Alexa.
The following are some examples of popular machine learning real-world applications:
Image Recognition
It is one of the most popular ML applications. It addresses individuals, objects, digital images, and places. Furthermore, automatic friend tagging is recognized in cases of face detection and image recognition.
Speech Recognition
We offer a voice search option when using Google; it falls under speech recognition and is a well-known machine learning program.
Online Fraud Detection
ML is developing secure and safe online transactions by addressing fraud. During online marketing, fraudulent transactions may occur in different ways, like fake identification documents, false online personas, and money theft transactions.
To overcome this, ML helps us assess whether marketing is genuine or fraudulent.
The result of each genuine transaction is converted into a set of hash values, and these values are then utilized as input for subsequent rounds.
Due to the distinct pattern for every valid transaction, it helps identify fraud and improves the safety of our online purchases.
Stock Market Trading
Frequently, machine learning is used in stock market trading. Because there is always a chance that share prices may go up and down, the long-short-term memory neural network in ML is used to anticipate stock market patterns.
Traffic Prediction
Google Maps helps us plan trips to new places because it predicts traffic and shows us the best way to get there.
To predict traffic conditions, like whether it’s going to be clear, actually moving, or congested, it employs two techniques:
- The Google Maps app, as well as sensors’ real-time determination of the vehicle’s location,
- It took an average amount of time on similar days in the past.
Every user of Google Maps contributes to its development. It accepts data from the user and sends it back to the server to improve speed.
Once we’ve looked at its applications, let’s thoroughly grasp deep learning’s operation and how it varies from machine learning and AI.
How Does Machine Learning Work?
There are three essential components to the ML algorithm learning process.
A Decision Process
Machine learning algorithms seek to identify patterns in data and use this understanding to predict future events. How does the model produce predictions, exactly?
The good news is that this process, which involves identifying a pattern in incoming data (whether labelled or unlabelled) and using it to produce outcomes, is relatively simple.
An Error Function
The goal of the machine learning model is to contrast its predictions with the actual results. The goal is to determine whether one is learning on the correct path. This establishes the model’s accuracy and suggests ways to enhance the model’s training.
A Model Optimization Process
The ultimate goal of the model is to make better predictions, which means that the difference between the actual outcome and the model estimate needs to be as small as possible.
By regularly changing the weights, the model must better suit the training data samples. The method runs in a loop, assessing and improving the output while updating the weights until the model’s accuracy is maximized.
Source: Google Cloud Tech
What is Deep Learning?
DL is a subcategory of ML that uses algorithms and teaches systems to do what comes naturally to humans. Deep learning systems may use large volumes of both organized and unstructured information. At the core of deep learning, artificial neural networks give machines the ability to think.
The main differentiation between DL and ML is the procedure for nurturing data into the system. While ML algorithms often need organized input, deep learning networks include multiple tiers of artificial neural networks.
A primary neural network seems like this:
A network input layer gets data from the network and practices it. The hidden layer is used to find any hidden properties in the data. The output unit ultimately delivers the required outcome.
An example of a neural network that uses large amounts of unlabelled visual information is shown below. Based on this information, the network algorithm is trained to determine whether an individual has diabetic retinopathy.
Let’s look at deep learning in more detail now that we understand it.
Types of Deep Learning
CNN (Convolutional Neural Network): It is a crucial type of DL that is highly practiced for image assessment.
RNN (Recurrent Neural Network): It uses sequential data for developing the models. It is highly significant to retain historical data in the system.
Generative Adversarial Network (GAN): It is an algorithmic design that produces new, artificial cases of data that may be misinterpreted for actual data using two neural networks. A GAN that learns from images might come up with new ideas that at least seem plausible to humans.
Deep Belief Network (DBN): It comprises many layers of unknown variables known as hidden units. Despite the units not being linked, each layer is interconnected. You can also consult a website development company to implement the DL effectively.
Applications of Deep Learning
The typical individual may find deep learning applications sad, but those who have had the chance to learn about the machine learning sector know the firm’s profound global influence. For instance, deep learning is tackling and researching human challenges in various sectors.
You will be amazed by the list of applications for deep learning and the explanations that follow.
Healthcare
The healthcare sector is using deep learning. Computers can now detect illnesses and identify their causes due to a deep understanding. It is often used with medical imaging for medical science, drug development, and detecting severe disorders, including cancer and diabetic retinopathy.
Picture Colouring
Image colouring has been greatly enhanced using deep learning. A grayscale image is used as the input for picture colorization, which produces a coloured image as a result. A
n example of an image colorization model is ChromaGAN. An adversarial model that frames a generating network and incorporates perceptual and semantic knowledge of class distributions and colour allows the web to learn to colorize.
Music Production
A machine may start making music independently after learning the notes, structures, and patterns of music. Deep learning-powered generative models like WaveNet may be used to produce raw audio. The extended short-term memory network aids the automatic creation of music.
The Python Music21 toolkit is used in computer-aided musicology. We can teach a system to make music by teaching it the fundamentals of music theory, making musical samples, and learning about music.
Image Captioning
The process of producing a written description for an image It uses computer vision to comprehend the content of the picture and a language model to translate that knowledge into the appropriate sequence of words.
When you submit a picture or enter the URL of any image, Microsoft’s caption bot will show a textual description for the image.
How Does Deep Learning Work?
- Calculate weighted sums first.
- The activation function gets the total computed weight as input.
- The activation function determines whether or not the cell might fire by taking the “weighted sum of input” as its input and adding a bias.
- The output layer displays the anticipated results.
- The prediction model and the actual output are contrasted. After learning, the model applies the training algorithm technique to increase the neural network’s effectiveness. The elimination problem helps reduce the error rate.
The instance below reads the license registration number using deep learning and neural networks.
Many nations use this technique to catch fast and maliciously operating vehicles.
Examples of Artificial Intelligence vs Machine Learning vs Deep Learning
To better understand how these strategies are used, let’s look at a few real-world examples.
Examples of AI
An excellent example of what artificial intelligence has made possible is self-driving cars.
A self-driving car is a device that develops driving abilities similar to those of humans. Because it currently needs human assistance, it could not be what some regard as “machine intelligence.”
However, it does a perfect job of imitating human intellect by navigating the routes and making significant judgments using facial recognition.
A few further instances of artificial intelligence are:
- Robots used in manufacturing and virtual assistants can learn about your preferences.
- Safety gadgets that identify and classify faces
- Smart home technologies that could comprehend what you’re talking about and provide contextual replies
Examples of Machine Learning
A well-known use of machine learning is image recognition. Consider how Facebook can recognize your contacts or how some applications might make purchase recommendations based on the contents of a photo you’ve uploaded.
During the training phase of this program, a large amount of raw data in the form of random pictures is fed to a machine learning system. Every time the system successfully connects two distinct photos, it is rewarded using a reinforcement learning approach.
Machine learning algorithms can eventually identify individuals and objects in photos reasonably accurately.
Other instances of machine learning apps include:
- Software for speech recognition
- Predictive analytics
- Translation tools
Examples of Deep Learning
Without deep learning, it will be impossible to create virtual assistants such as Siri. A virtual assistant is a software program that can do the difficult job of conversing with a person.
In contrast, displaying natural language comprehension analyses fresh inputs and determines what appropriate answers can be given each time.
Deep learning is also used to produce the following things:
- Utilizing MRI image data for automated disease detection
- goods that automatically color photographs by first detecting the stuff in them
- The discovery of novel pharmaceuticals by analyzing patterns in already available pharmaceutical products
- Decision support systems like those employed by Netflix and Amazon
What is the Distinction Between AI, ML, and DL?
The following table shows the difference between machine learning, deep learning, and artificial intelligence:
Artificial Intelligence | Machine Learning | Deep Learning |
The text means that artificial intelligence is being activated or stimulated. | It is the method used to get machines to behave autonomously in programming. | It entails employing artificial neural networks to tackle challenging issues. |
data science. | It is part of data science and AI. | It is part of data science and AI. |
It strives to develop machines that can think just like humans. | It aims to teach computers to learn from data so that they might solve issues. | It seeks to create neural networks that automatically look for patterns to recognize features. |
Applications of Artificial Intelligence
1. Artificial intelligence-based robots, including Sophia and Aibo; self-driving services, including Google’s Waymo. |
Applications of machine learning
1. Estimating sales for various items |
Applications of Deep Learning
1. Object identification |
Conclusion
Among the most popular 5th-generation techniques, the world is being revolutionized by AI and its subsets, ML and DL. AI enables the development of intelligent systems and gives machines cognitive capabilities.
Additionally, machine learning helps computers learn through experience without human interaction and equips them with the ability to learn from and anticipate outcomes using supplied data.
Deep learning is a development in AI that combines several artificial neural network layers to provide excellent results for various issues, including text and picture recognition.
As a result, after reading this material, you can state that most individuals do not struggle to distinguish between these phrases. After reading this subject, you should feel confident that you can distinguish between machine learning, deep learning, and artificial intelligence.
FAQs About AI, Machine Learning, and Deep Learning
Your most commonly asked questions have answers here.
1. How does Machine Learning differ from AI?
The vast field of AI aims to make it possible for a system to replicate human intellect. ML is an area of artificial intelligence that aims to develop algorithms and techniques that could assist software in learning from and seeing trends in massive amounts of data.
2. Can I learn deep learning without ML?
No. To emphasize deep learning, you must study machine learning.
3. Is deep learning the same as AI?
The field of deep learning may be categorized as an AI discipline. For data analysis and understanding, the discipline employs layers of learning networks.
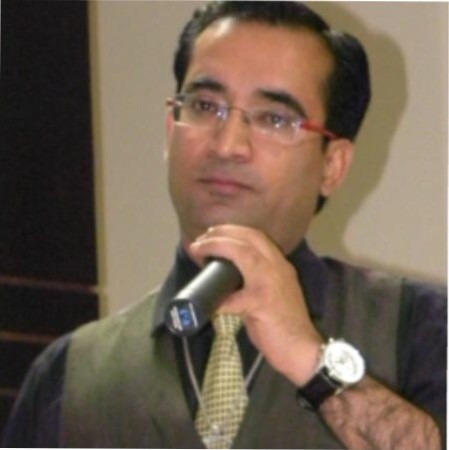
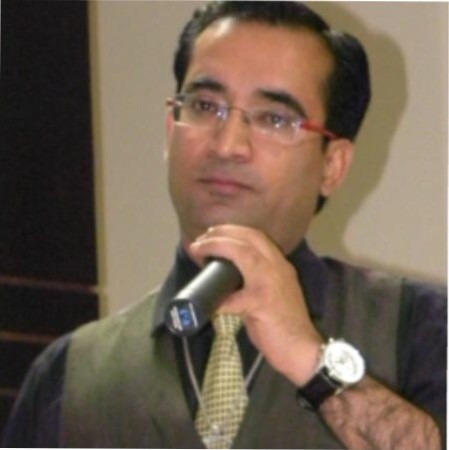
Naveen Khanna is the CEO of eBizneeds, a company renowned for its bespoke web and mobile app development. By delivering high-end modern solutions all over the globe, Naveen takes pleasure in sharing his rich experiences and views on emerging technological trends. He has worked in many domains, from education, entertainment, banking, manufacturing, healthcare, and real estate, sharing rich experience in delivering innovative solutions.